Certainly uncertain – living in a post-bright line world
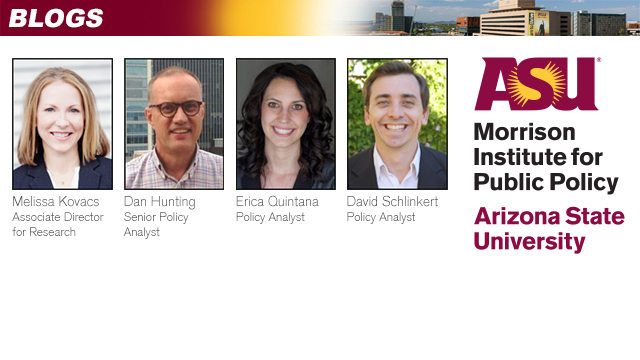
Melissa Kovacs, Dan Hunting, Erica Quintana, and David Schlinkert
May 28, 2019
Background
After 177 years of silence, the American Statistical Association (ASA) issued a statement last month suggesting a change to one of the fundamental tenants of research: the p-value is no longer the guiding bright line between certainty and uncertainty.
Many disciplines use a “p is less than 0.05” threshold to note when research results are deemed “significant.” This has served as a convenient statistical shorthand for many years: if a p-value is less than 0.05, then an effect exists in the data. If it is greater than 0.05, no significant effect is present. Clients, stakeholders, editors, and policymakers have become accustomed to searching for the p-value result, and at times make decisions about a study’s validity based solely on whether the value is over or under 0.05. The p-value and its rules provide structure and certainty, helping researchers and practitioners make consistent decisions in spite of uncertainty in research.
Yet the real world is not so straightforward. Statistical significance does not suddenly spring forth when the magic 0.05 barrier is crossed. Findings and effects should be determined by many factors, not just the p-value. For example, important findings may well have p-values over the 0.05 threshold.
A world with fewer bright lines will increase ambiguity and create a gradient of certainty instead of a hard-lined, yes or no rule. After all, how big of a difference is there between a p-value of .049 and .051?
What does a post-p-value world mean for public policy researchers?
Like other policy researchers, Morrison Institute has made nuanced discussion of policy issues a priority for decades. Policy researchers have long been aware that complex problems are rarely amenable to simple yes/no judgments. We’re comfortable exploring the subtleties of data and clearly describing our findings not only with raw numbers, but also with incisive writing and informative graphics.
Without a heavy reliance on p-values, deciphering statistical findings will require more attention to the details and nuances of data. To explain these subtleties, effective data communications will continue to be important in public policy research. We will need to have longer conversations with stakeholders regarding the meaning of research results. There will also be an increased reliance on data visualizations.
Data visualizations can show more nuance than a text table of results. By visualizing results, research consumers can see how similar or dissimilar the outcomes are in an evaluation between research participants and non-participants. Visualizations can also show, in picture form, multiple metrics about a research outcome in one snapshot.
As a policy community, we will have to pivot towards a new common language to convey when research results are relevant.
What will this mean for policy reporting?
Although public policy research employs sophisticated statistical techniques at times, it more often uses descriptive statistics. These descriptive techniques provide informative policy options without the use of p-values.
At Morrison Institute, while our researchers all have considerable skills with statistics, we don’t typically report p-values in our reports. When we do conduct statistical techniques on data, we report multiple statistical measures, not just the p-value. We discuss sample size along with parameter size, strength, and direction. Policy researchers are trained to think about all of these statistical signals and digest them for our audience. We are committed to straightforward, transparent analysis, talking about how we arrived at our conclusions rather than refer to a statistical black box that magically reports results.
Policy researchers are typically providing policy options that are grounded in strength and notability, without solely relying on frequentist statistical techniques and the bright line of a p-value.
What will the field do without a bright line?
Policy researchers are inherently interested in social science nuance. We are accustomed to thinking about context around statistical results, and not just the results themselves.
Regardless of how slowly or quickly a statistical culture shift occurs, we’ll continue to fully consider multiple statistical signals when reporting research findings and potential policy options.